Autoencoders and Regularization - Learn and Implement
In this course, you'll explore how Autoencoders compress, denoise, and derive valuable features from data. You'll also delve into regularization to curb overfitting and boost model generalizability. Autoencoders play roles in image enhancement, anomaly spotting, recommendation engines, and generative modelling. Meanwhile, regularization is essential in nearly every machine-learning endeavor. This training will equip you to address practical challenges across various applications.
4.4 (49 Reviews)
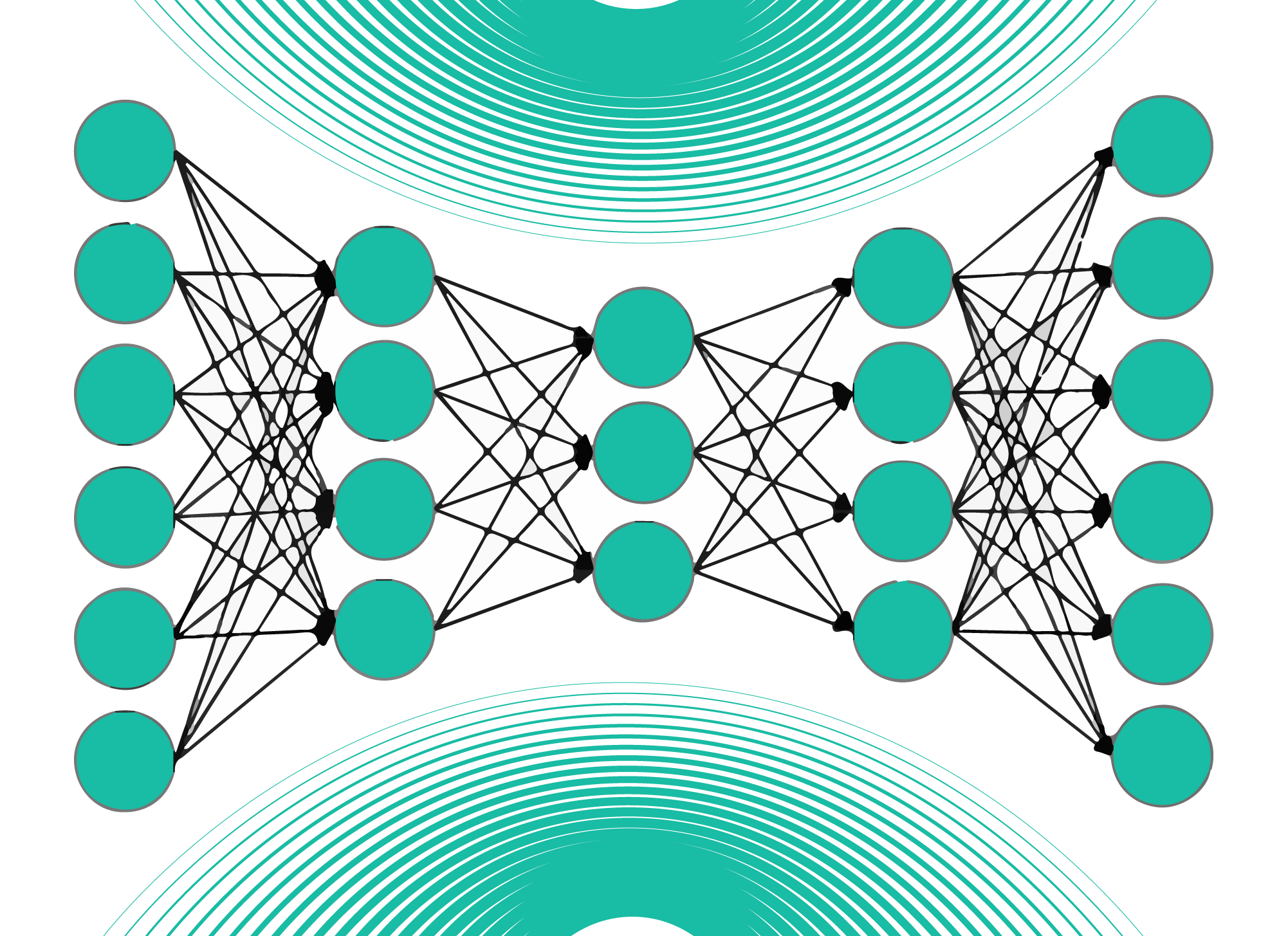
Language
- English
Topic
- Artificial Intelligence
Enrollment Count
- 302
Skills You Will Learn
- Artificial Intelligence, Data Science, Keras, Deep Learning
Offered By
- IBMSkillsNetwork
Estimated Effort
- 3 hours
Platform
- SkillsNetwork
Last Update
- July 1, 2025
IBM has a special offer for watsonx.ai a studio for new foundation models, generative AI and machine learning. To take advantage of this offer visit watsonx.ai homepage.
Course Syllabus
- Regularization
- Autoencoders
- Variational Autoencoders
Recommended Skills Prior to Taking this Course
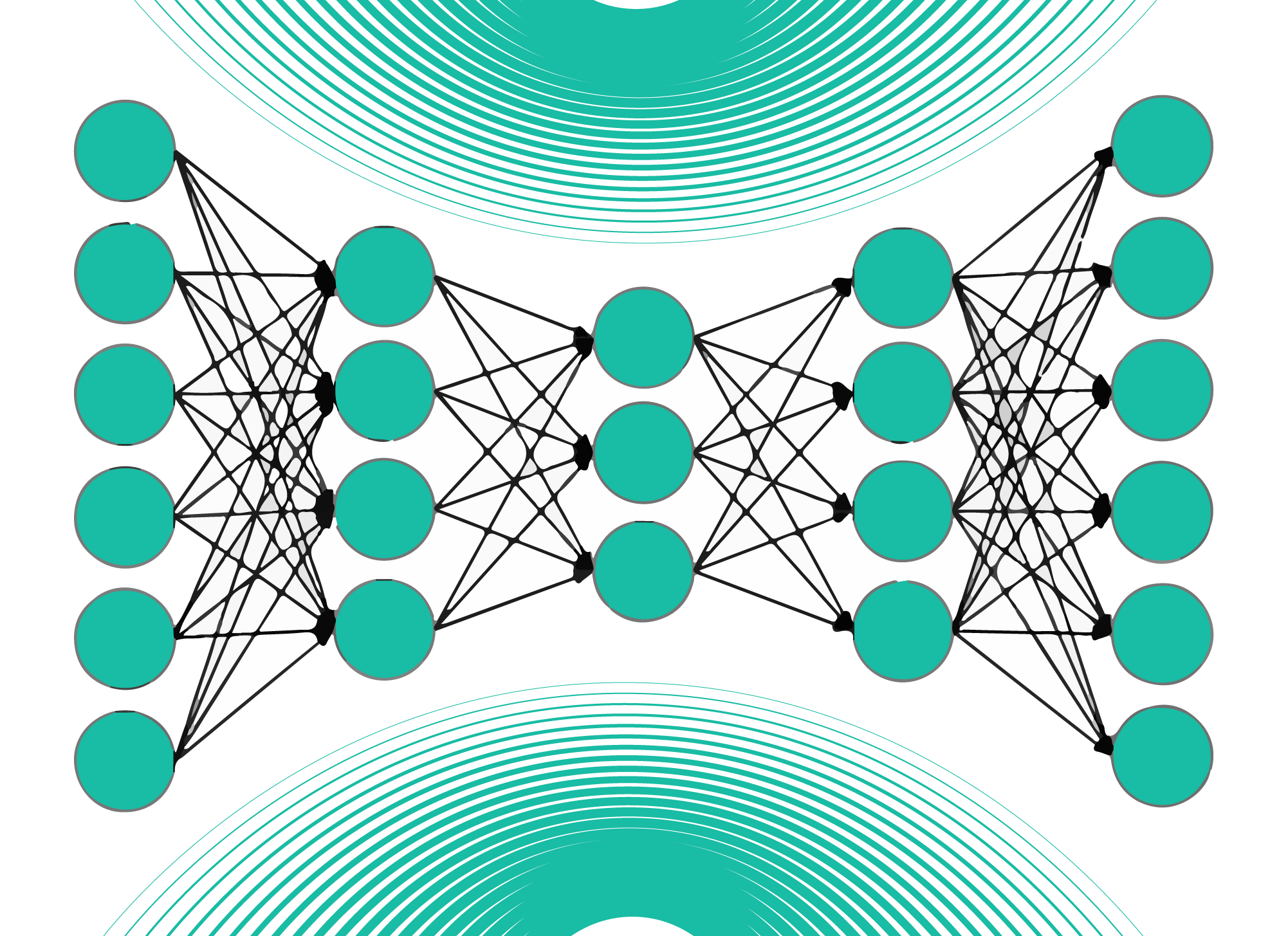
Language
- English
Topic
- Artificial Intelligence
Enrollment Count
- 302
Skills You Will Learn
- Artificial Intelligence, Data Science, Keras, Deep Learning
Offered By
- IBMSkillsNetwork
Estimated Effort
- 3 hours
Platform
- SkillsNetwork
Last Update
- July 1, 2025
Instructors
Artem Arutyunov
Data Scientist
Hey, Artem here! I am excited about answering new challenges with data science, machine learning and especially Reinforcement Learning. Love helping people to learn, and learn myself. Studying Math and Stats at University of Toronto, hit me up if you are from there as well.
Read moreJoseph Santarcangelo
Senior Data Scientist at IBM
Joseph has a Ph.D. in Electrical Engineering, his research focused on using machine learning, signal processing, and computer vision to determine how videos impact human cognition. Joseph has been working for IBM since he completed his PhD.
Read moreKopal Garg
Data Scientist Intern at IBM
I am a Data Scientist Intern at IBM, and a Masters student in Computer Science at the University of Toronto. I am passionate about building data science, and machine learning-based systems for improving various aspects of life.
Read moreRoxanne Li
Data Scientist at IBM
I am an aspiring Data Scientist at IBM with extensive theoretical/academic, research, and work experience in different areas of Machine Learning, including Classification, Clustering, Computer Vision, NLP, and Generative AI. I've exploited Machine Learning to build data products for the P&C insurance industry in the past. I also recently became an instructor of the Unsupervised Machine Learning course by IBM on Coursera!
Read moreContributors
Roodra Kanwar
Data Scientist at IBM
I am a data scientist by day, superhero by night. Psych! I wish I was that cool. Only the former part is true which is still pretty cool! I believe in constant learning and it is an essential part of being a productive data enthusiast. I am also pursuing my masters in computer science from Simon Fraser University specializing in Big Data. Moreover, knowledge is transfer learning (pun intended!) and what I have gained, I plan on reflecting it back to the data community.
Read more