Efficient models: reduce dimensionality with LDA in Python
Understand and implement Linear Discriminant Analysis (LDA), one of the best ML methods for dimensionality reduction in classification tasks. Dimensionality reduction is a fundamental machine learning technique that is frequently used to improve the performance of prediction models, interpretability, and data visualization. This easy-to-follow, hands-on project walks you through understanding LDA, when it's most useful, and how to implement this dimensionality reduction technique using Python.
4.8 (5 Reviews)
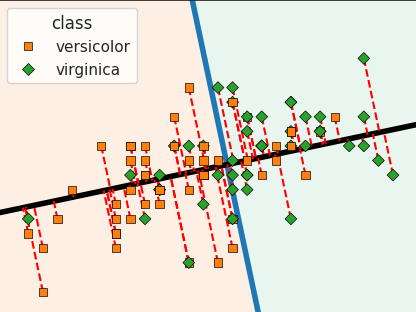
Language
- English
Topic
- Machine Learning
Skills You Will Learn
- Python, Machine Learning, Data Science, Numpy, Pandas, sklearn
Offered By
- IBMSkillsNetwork
Estimated Effort
- 45 minutes
Platform
- SkillsNetwork
Last Update
- May 13, 2024
A Look at the Project Ahead
- Learn how LDA works
- Plot the LDA decision boundary for a binary classification problem
- Use LDA for classification
- Use LDA for dimensionality reduction
- Learn how to implement LDA using Python
What You'll Need
While having a basic grasp of statistics, data science, and/or machine learning is helpful for following along, it's not strictly required. The project is designed to be as accessible as possible to a general audience, with explanations primarily delivered in a graphical and intuitive manner. Whether you're a beginner just starting out, or a seasoned professional looking for a refresher on LDA, this hands-on project is for you!
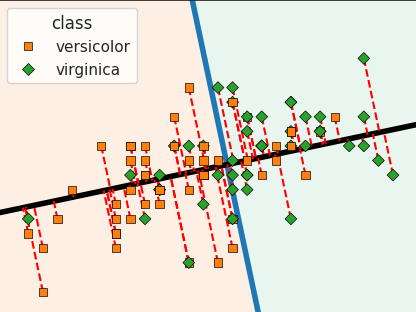
Language
- English
Topic
- Machine Learning
Skills You Will Learn
- Python, Machine Learning, Data Science, Numpy, Pandas, sklearn
Offered By
- IBMSkillsNetwork
Estimated Effort
- 45 minutes
Platform
- SkillsNetwork
Last Update
- May 13, 2024
Instructors
Eda Kavlakoglu
Program Director, Technical Content at IBM
Marketing leader with a technical background in data science.
Read moreWojciech "Victor" Fulmyk
Data Scientist at IBM
As a data scientist at the Ecosystems Skills Network at IBM and a Ph.D. candidate in Economics at the University of Calgary, I bring a wealth of experience in unraveling complex problems through the lens of data. What sets me apart is my ability to seamlessly merge technical expertise with effective communication, translating intricate data findings into actionable insights for stakeholders at all levels. From modeling to storytelling, I bring a holistic approach to data science. Leveraging machine learning algorithms, I construct predictive models tailored to both real-world challenges as well as old, well-understood problems. My knack for data-driven storytelling ensures that the insights uncovered resonate with both technical and non-technical audiences. Open to collaboration, I'm eager to take on new challenges and contribute to transformative data-driven endeavors. Whether you seek to extract insights, enhance predictive models, or explore untapped potential within your datasets, I'm here to help. Feel free to connect to me via my LinkedIn profile. Let's learn from each other!
Read moreContributors
Lucy Xu
Data Scientist
I am a Data Scientist Intern at IBM. I am also currently in my fourth year at the University of Waterloo studying Statistics with a minor in Computing.
Read more