Image Segmentation with Mean Shift Clustering
From image segmentation to anomaly detection, Mean Shift Clustering offers a versatile and powerful solution for a wide range of data analysis challenges. It is no ordinary algorithm - it's a dynamic and non-parametric technique that can navigate through complex data terrains, finding density peaks that lead to clusters of diverse shapes and sizes and more. In this guided project, you will learn how to identify complex patterns, clusters, and subgroups in your datasets and use it for image segmentation.
4.5 (181 Reviews)
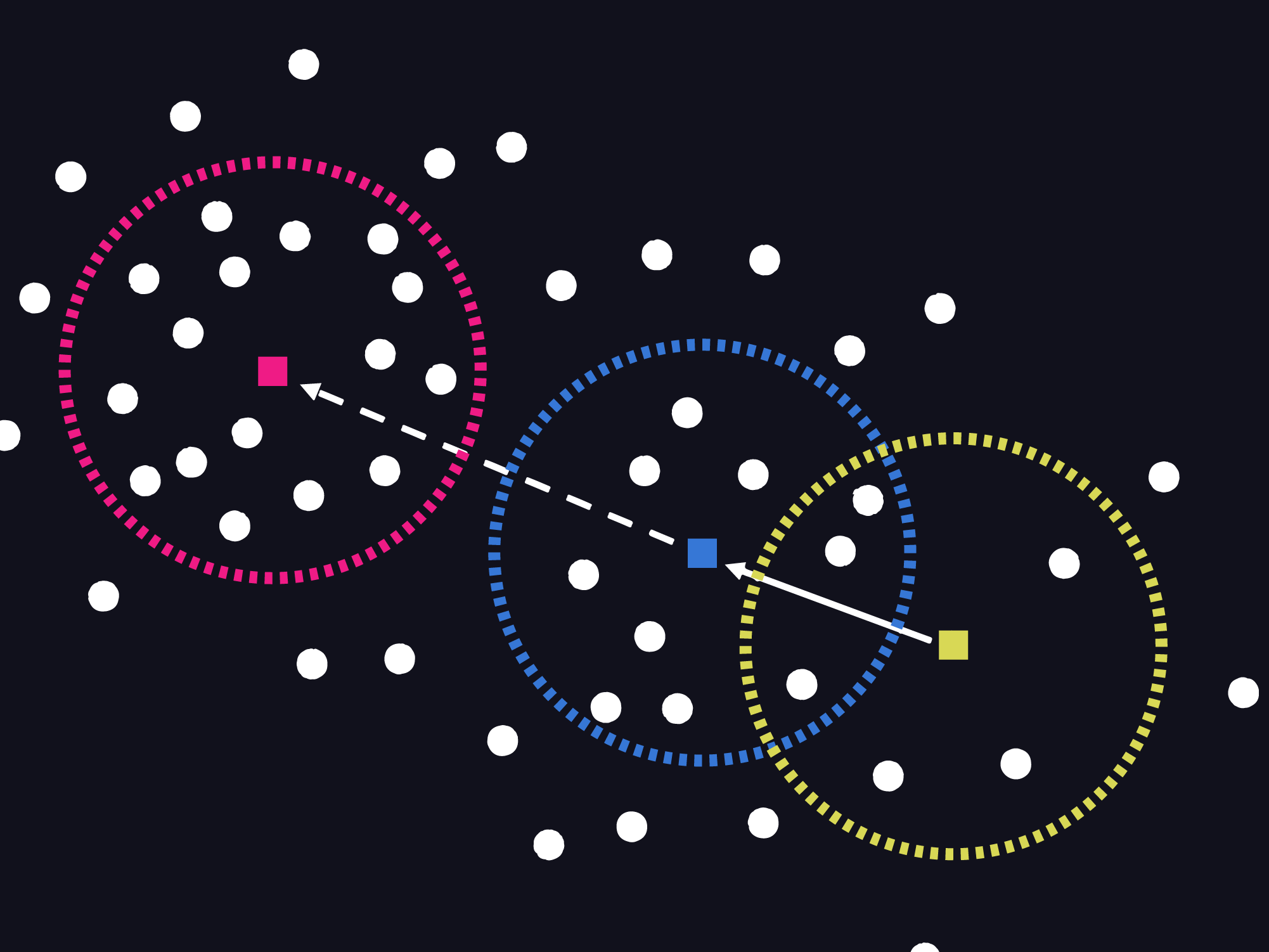
Language
- English
Topic
- Data Science
Enrollment Count
- 1.32K
Skills You Will Learn
- Data Science, Clustering, Python
Offered By
- IBMSkillsNetwork
Estimated Effort
- 30 minutes
Platform
- SkillsNetwork
Last Update
- May 29, 2025
In the first part of this guided project, we will focus on the image segmentation, which is used in many object detection and tracking systems, as it makes it easier to detect the contour of each object. In the second part, we will show how to use the Mean Shift Clustering to classify the survivors rates of the Titanic, the most famous shipwreck in history. Based on the passengers' features (e.g. age, ticket class, fare, etc.) we will classify the passengers into clusters with different survival probabilities.
Who should participate?
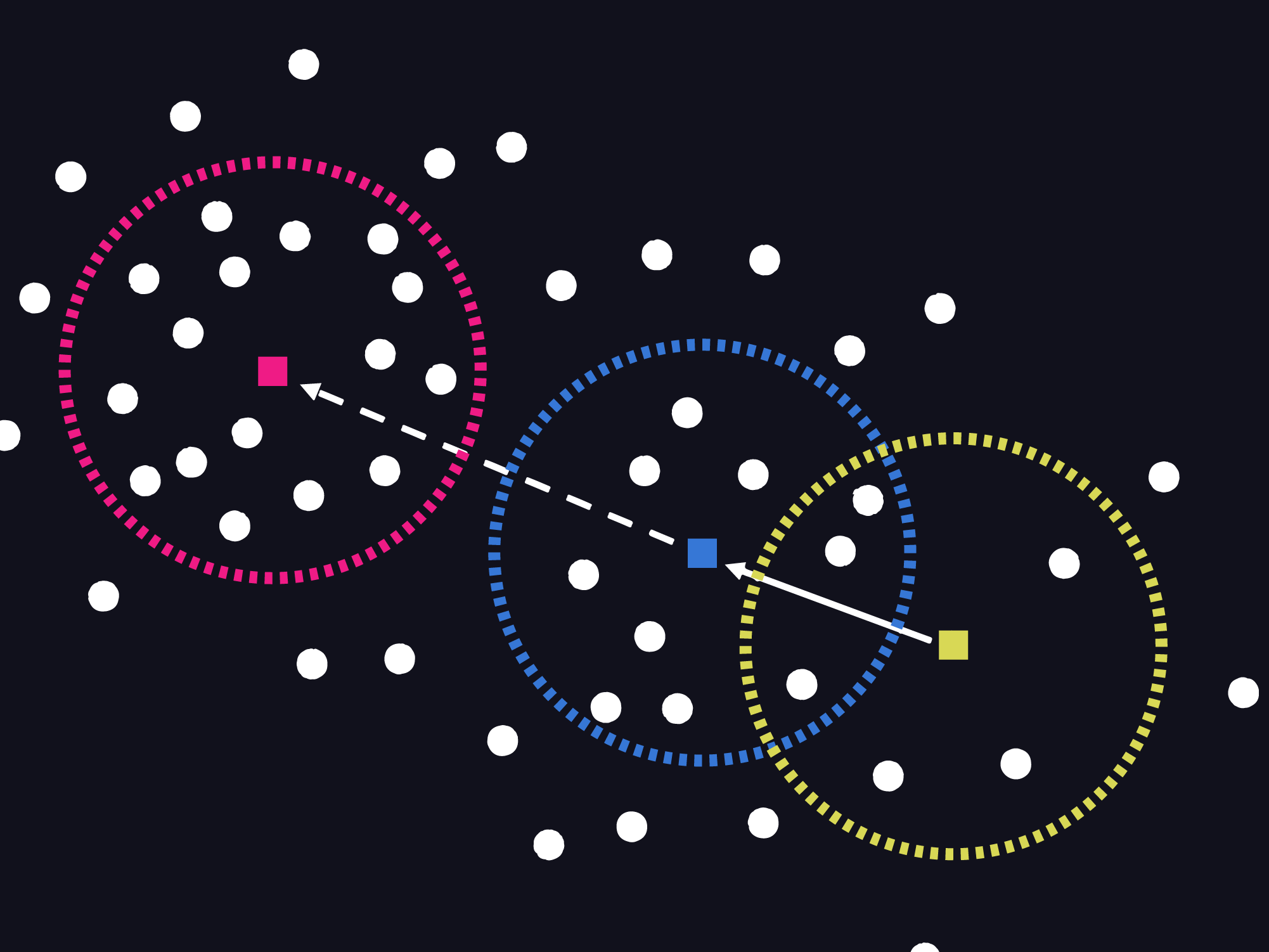
Language
- English
Topic
- Data Science
Enrollment Count
- 1.32K
Skills You Will Learn
- Data Science, Clustering, Python
Offered By
- IBMSkillsNetwork
Estimated Effort
- 30 minutes
Platform
- SkillsNetwork
Last Update
- May 29, 2025
Instructors
Joseph Santarcangelo
Senior Data Scientist at IBM
Joseph has a Ph.D. in Electrical Engineering, his research focused on using machine learning, signal processing, and computer vision to determine how videos impact human cognition. Joseph has been working for IBM since he completed his PhD.
Read moreRoxanne Li
Data Scientist at IBM
I am an aspiring Data Scientist at IBM with extensive theoretical/academic, research, and work experience in different areas of Machine Learning, including Classification, Clustering, Computer Vision, NLP, and Generative AI. I've exploited Machine Learning to build data products for the P&C insurance industry in the past. I also recently became an instructor of the Unsupervised Machine Learning course by IBM on Coursera!
Read moreArtem Arutyunov
Data Scientist
Hey, Artem here! I am excited about answering new challenges with data science, machine learning and especially Reinforcement Learning. Love helping people to learn, and learn myself. Studying Math and Stats at University of Toronto, hit me up if you are from there as well.
Read moreContributors
Roodra Kanwar
Data Scientist at IBM
I am a data scientist by day, superhero by night. Psych! I wish I was that cool. Only the former part is true which is still pretty cool! I believe in constant learning and it is an essential part of being a productive data enthusiast. I am also pursuing my masters in computer science from Simon Fraser University specializing in Big Data. Moreover, knowledge is transfer learning (pun intended!) and what I have gained, I plan on reflecting it back to the data community.
Read more