Generative AI Advance Fine-Tuning for LLMs
Learn on
Advance your skills in fine-tuning language models with our course on Generative AI. You will explore reinforcement learning techniques, such as Proximal Policy Optimization (PPO) and Direct Preference Optimization (DPO), to enhance model security. Learn how to effectively use Hugging Face for instruction tuning. This course is designed for intermediate learners eager to enhance their AI expertise securely.
4.2 (73 Reviews)
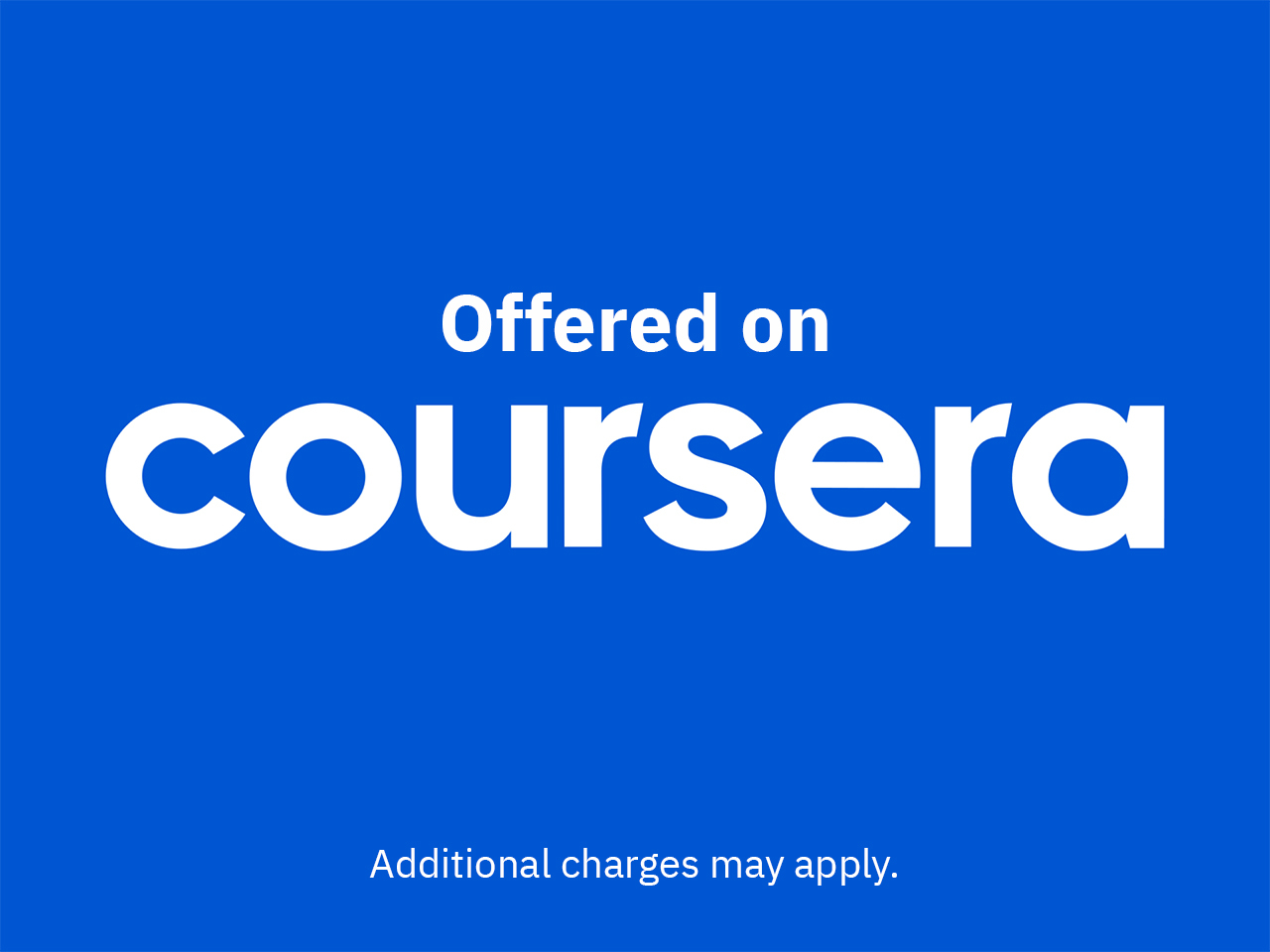
Language
- English
Topic
- Security
Industries
- Generative AI
Enrollment Count
- 5.77K
Skills You Will Learn
- Reinforcement Learning, Proximal Policy Optimization (PPO), Direct Preference Optimization (DPO), Hugging Face, Instruction Tuning
Offered By
- IBMSkillsNetwork
Estimated Effort
- 2 weeks/5hrs
Platform
- Coursera
Last Update
- April 30, 2025
- A solid understanding of basic Generative AI concepts and models.
- Experience with Python programming, particularly in AI/ML contexts.
- Familiarity with Hugging Face and reinforcement learning concepts.
Course 1: Generative AI and LLMs: Architecture and Data Preparation
Course 2: Generative AI Foundational Models for NLP & Language Understanding
Course 3: Generative AI Language Modeling with Transformers
Course 4: Generative AI Engineering and Fine-Tuning Transformers
- Understand and apply instruction-tuning techniques with Hugging Face.
- Develop and train reward models for LLMs.
- Implement proximal policy optimization (PPO) with Hugging Face.
- Leverage LLMs as policies in reinforcement learning contexts.
- Perform direct performance optimization (DPO) using Hugging Face.
- Hugging Face
- Python
- Reinforcement Learning Libraries
- Reward modeling
- Stay organized and keep track of deadlines.
- Regularly practice using the tools and technologies discussed in the course.
- Engage with the community and ask questions if you need help.
- Experiment with the examples provided to deepen your understanding.
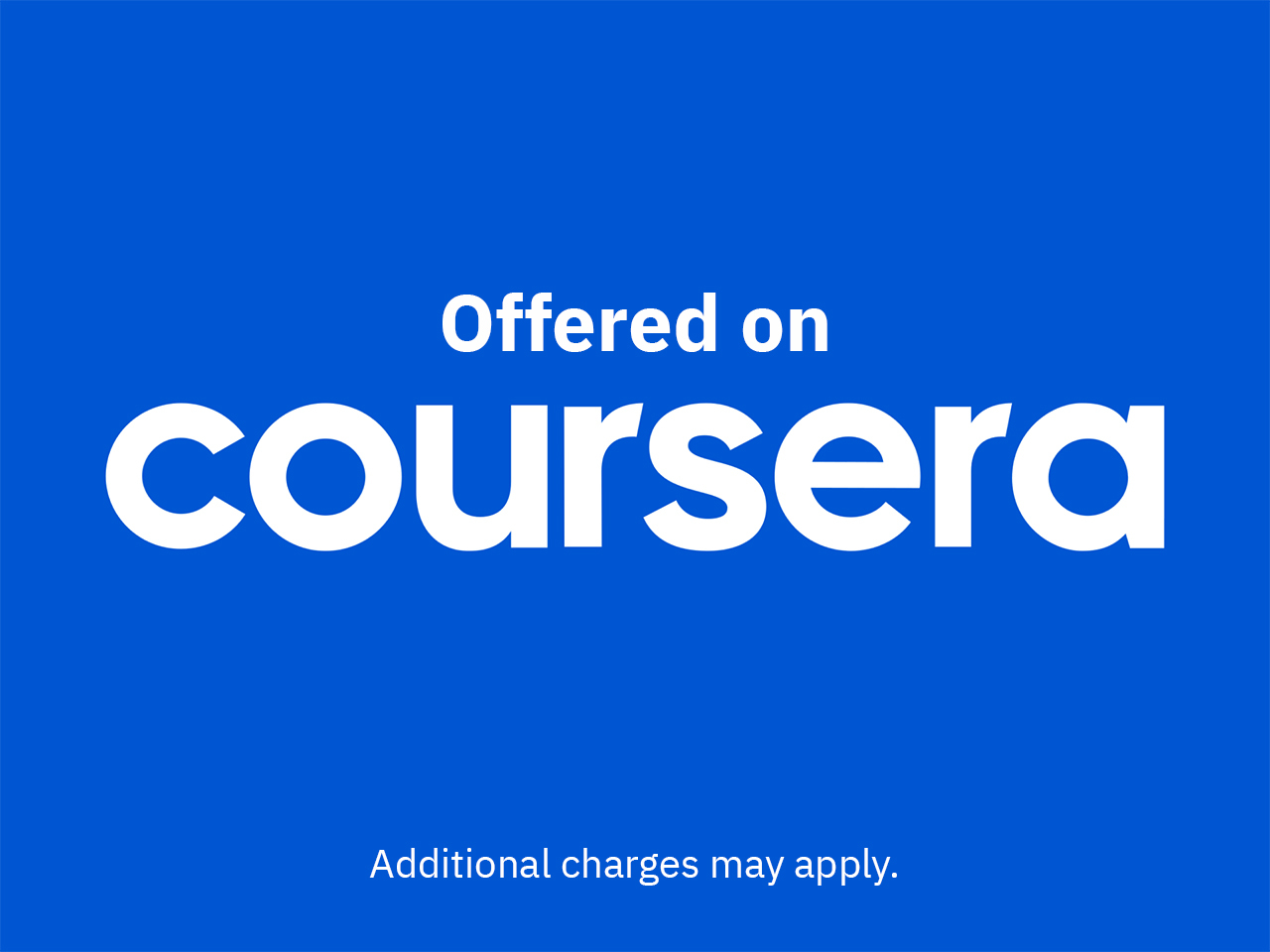
Language
- English
Topic
- Security
Industries
- Generative AI
Enrollment Count
- 5.77K
Skills You Will Learn
- Reinforcement Learning, Proximal Policy Optimization (PPO), Direct Preference Optimization (DPO), Hugging Face, Instruction Tuning
Offered By
- IBMSkillsNetwork
Estimated Effort
- 2 weeks/5hrs
Platform
- Coursera
Last Update
- April 30, 2025
Instructors
Joseph Santarcangelo
Senior Data Scientist at IBM
Joseph has a Ph.D. in Electrical Engineering, his research focused on using machine learning, signal processing, and computer vision to determine how videos impact human cognition. Joseph has been working for IBM since he completed his PhD.
Read moreRav Ahuja
Global Program Director, IBM Skills Network
Rav Ahuja is a Global Program Director at IBM. He leads growth strategy, curriculum creation, and partner programs for the IBM Skills Network. Rav co-founded Cognitive Class, an IBM led initiative to democratize skills for in demand technologies. He is based out of the IBM Canada Lab in Toronto and specializes in instructional solutions for AI, Data, Software Engineering and Cloud. Rav presents at events worldwide and has authored numerous papers, articles, books and courses on subjects in managing and analyzing data. Rav holds B. Eng. from McGill University and MBA from University of Western Ontario.
Read moreFateme Akbari
Data Scientist @IBM
I'm a data-driven Ph.D. Candidate at McMaster University and a data scientist at IBM, specializing in machine learning (ML) and natural language processing (NLP). My research focuses on the application of ML in healthcare, and I have a strong record of publications that reflect my commitment to advancing this field. I thrive on tackling complex challenges and developing innovative, ML-based solutions that can make a meaningful impact—not only for humans but for all living beings. Outside of my research, I enjoy exploring nature through trekking and biking, and I love catching ball games.
Read more