Supervised Machine Learning: Classification
Learn on
This course teaches the basics of Classification in Supervised Machine Learning, with a focus on using best practices for data sets with unbalanced classes. Ideal for aspiring data scientists. Prerequisites: Python, Data Cleaning, EDA, Calculus, Linear Algebra,
4.8 (412 Reviews)
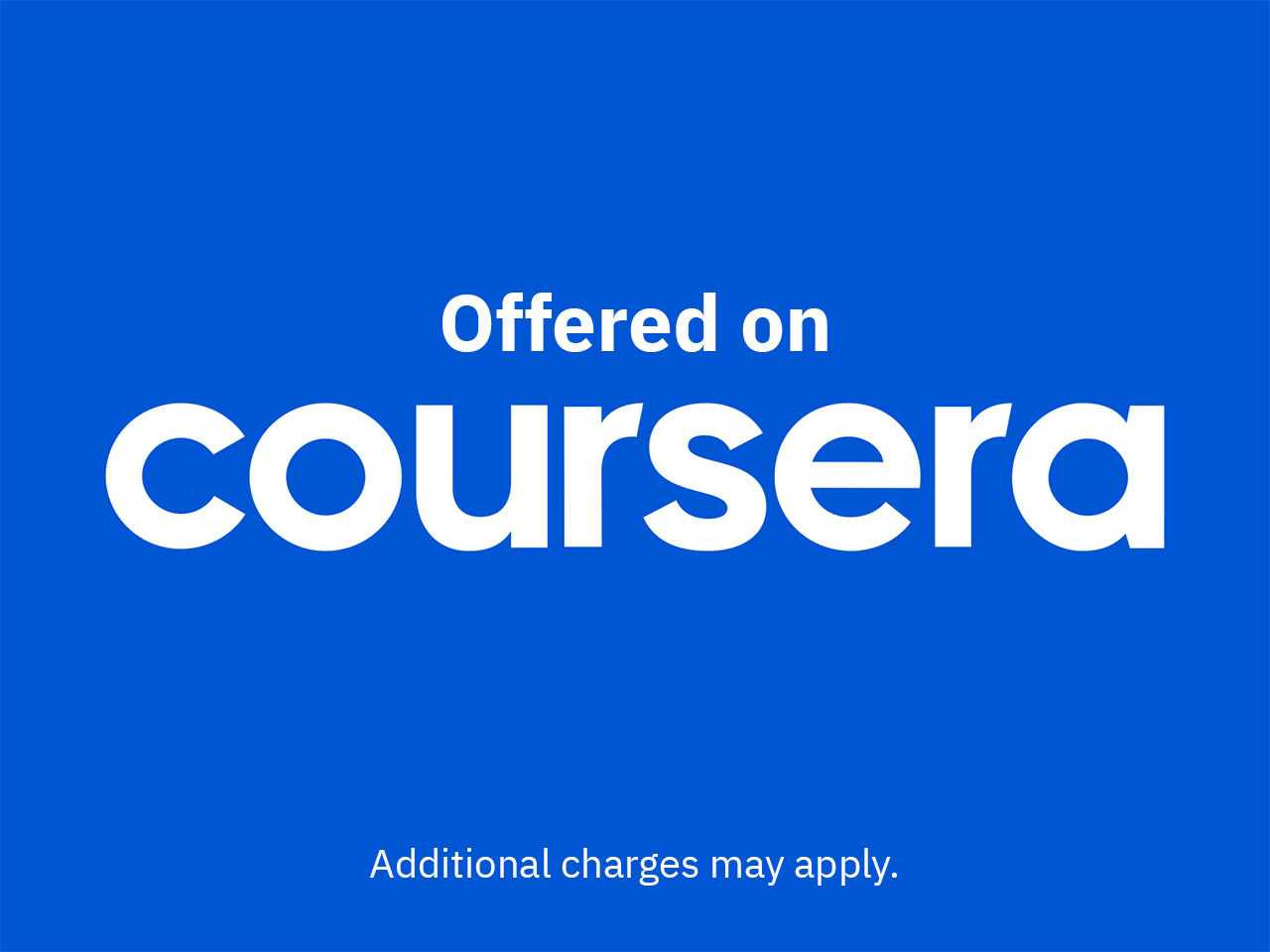
Language
- English
Topic
- Machine Learning
Enrollment Count
- 44.62K
Skills You Will Learn
- Machine Learning, Machine Learning Algorithms, Probability & Statistics, General Statistics, Theoretical Computer Science
Offered By
- IBMSkillsNetwork
Estimated Effort
- 5 weeks
Platform
- Coursera
Last Update
- June 26, 2025
By the end of this course you should be able to:
- Differentiate uses and applications of classification and classification ensembles
- Describe and use logistic regression models
- Describe and use decision tree and tree-ensemble models
- Describe and use other ensemble methods for classification
- Use a variety of error metrics to compare and select the classification model that best suits your data
- Use oversampling and undersampling as techniques to handle unbalanced classes in a data set
Who should take this course?
This course targets aspiring data scientists interested in acquiring hands-on experience with Supervised Machine Learning Classification techniques in a business setting.
What skills should you have?
To make the most out of this course, you should have familiarity with programming on a Python development environment, as well as fundamental understanding of Data Cleaning, Exploratory Data Analysis, Calculus, Linear Algebra, Probability, and Statistics.
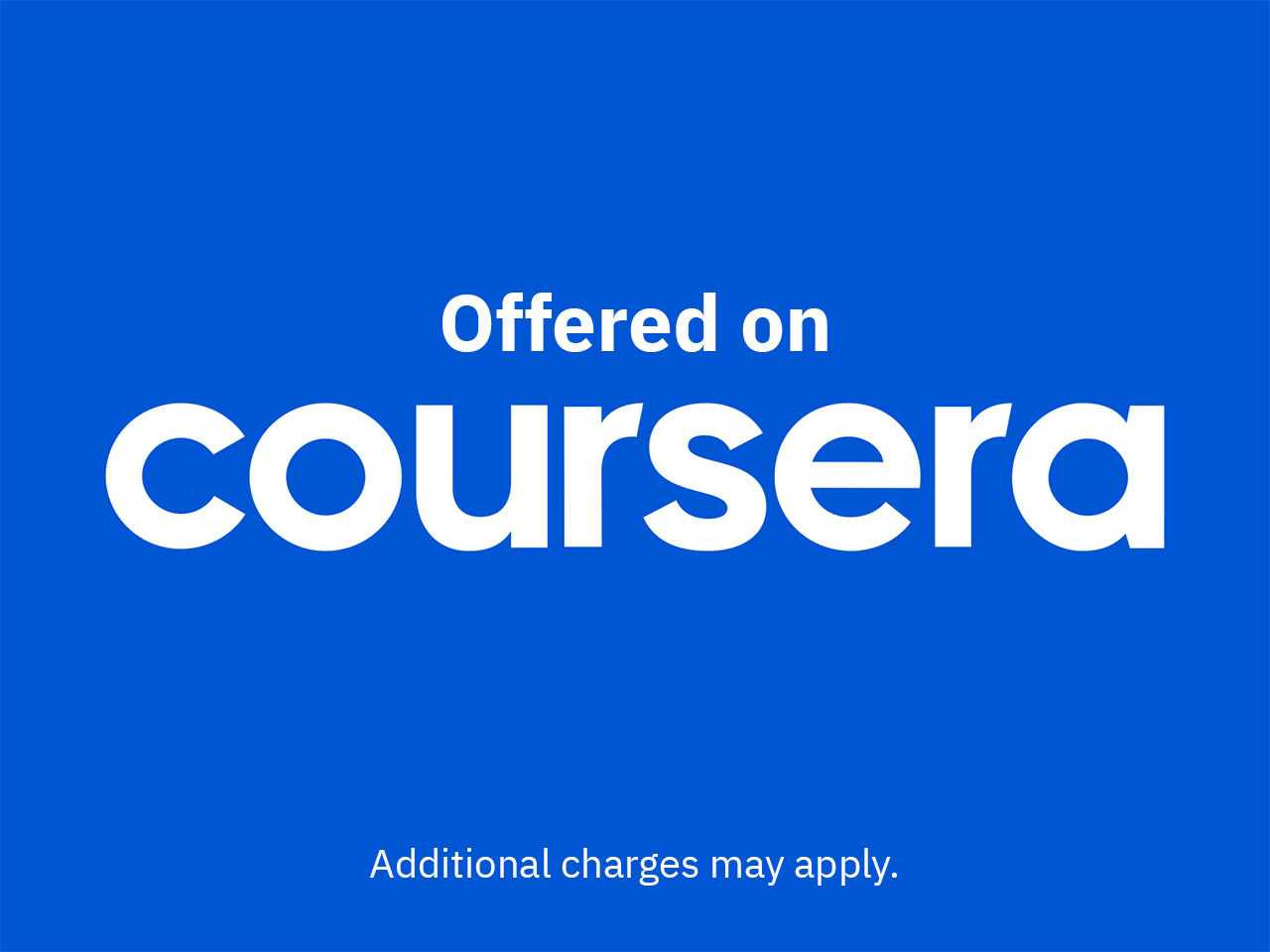
Language
- English
Topic
- Machine Learning
Enrollment Count
- 44.62K
Skills You Will Learn
- Machine Learning, Machine Learning Algorithms, Probability & Statistics, General Statistics, Theoretical Computer Science
Offered By
- IBMSkillsNetwork
Estimated Effort
- 5 weeks
Platform
- Coursera
Last Update
- June 26, 2025
Instructors
Joseph Santarcangelo
Senior Data Scientist at IBM
Joseph has a Ph.D. in Electrical Engineering, his research focused on using machine learning, signal processing, and computer vision to determine how videos impact human cognition. Joseph has been working for IBM since he completed his PhD.
Read moreSvitlana Kramar
Data Scientist
I’m a passionate data science educator whose goal is to learn by teaching innovative data science tools that can improve our day-to-day tasks and our quality of life. My interests are in Natural Language Processing: text classification, summarization, and generation. Research can take a long time because there are a lot of resources and new opinions posted every day. Having tools to summarize and extract the information can save a lot of time. I hope we can all learn, approve, and apply the data science tools to cut down on the repetitive and tedious tasks, to make more informed decisions in life, to differentiate fake from real, and to open communication spaces to language-diverse or hearing-impaired audiences. The applications are limitless! My personality: I am a foodie and I love cooking and learning different cuisines. I also love travelling and connecting with people by learning a little bit of their language, about their food and music. I hold Data Science and Analytics master’s degree, specializing in Machine Learning, from University of Calgary.
Read more